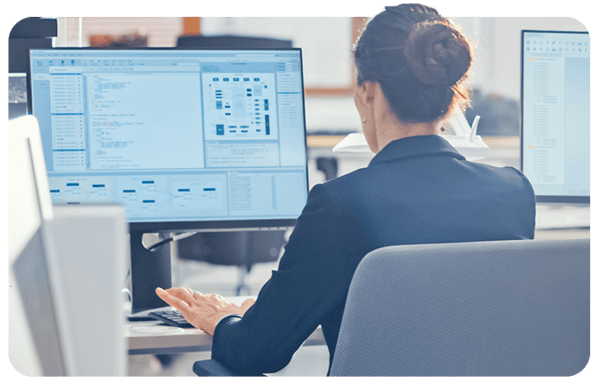
Intraday Performance Analysis: A New Perspective on Real-Time Staffing Adjustments
Introduction
Imagine it’s midday in your contact center. A few more agents than expected have called out sick, leaving you with less coverage than planned. You’re already making adjustments when, suddenly, call volumes surge far beyond the forecast. The carefully scheduled training and coaching sessions you worked so hard to plan are canceled. Agents’ handling times spike as they manage the back-to-back influx of calls. Despite your best efforts, service levels plummet. Calls queue up, and the manual adjustments your workforce management team is making just aren’t fast enough to keep pace.
This scenario is all too common in contact centers. But what if you already had the data at your fingertips to prevent these issues? Prior Day Performance (PDP) data holds the key to identifying the inefficiencies baked into traditional staffing models, which rely too heavily on fixed forecasts and pre-planned schedules. In this article, we’ll explore how analyzing PDP data can help reveal these shortcomings and why adopting more dynamic, real-time staffing models like Erlang-O is crucial for optimizing both service levels and operational costs.
The Challenge with Traditional Staffing Models
Most contact centers still rely on legacy staffing models like Erlang-C and Erlang-A. These models are built on the assumption that staffing levels can be perfectly matched to forecasted demand, aiming for a “net-zero” approach where the number of agents scheduled equals the predicted number of calls. However, this rigid method leaves little room for the unexpected.
In practice, slight fluctuations—such as a few agents calling in sick or a sudden uptick in customer demand—can cause significant disruption. Understaffing can lead to long customer wait times and missed service level targets, while overstaffing results in idle agents and increased labor costs.
Traditional models are simply not designed to handle the natural variance and volatility that occur during the day. They leave contact centers vulnerable to inefficiencies and unpredictable outcomes, creating what we call a fragile capacity plan.
Unlocking the Power of PDP Data for Real-Time Insights
Contact centers collect vast amounts of data daily, particularly through Prior Day Performance (PDP) reports. These reports provide detailed metrics on staffing levels, service levels, and call volumes across granular intervals. The challenge isn’t the availability of data, but how to transform it into insights that expose inefficiencies and guide smarter decision-making.
PDP data offers a clear view of how well staffing levels matched actual demand. The goal isn’t just to analyze the data, but to tell a story: a story of where traditional staffing models fall short and how new approaches can bridge the gap.
PDP data shows exactly where and when your contact center is under or overstaffed in each interval, providing valuable insights into operational inefficiencies. Understaffing leads to service level risks, while overstaffing drives unnecessary costs. However, analyzing this data across hundreds or thousands of queues over weeks of interval data can be daunting. This is where traditional models like Erlang-C or Erlang-A fall short. By recognizing clear patterns within PDP data, WFM teams can make a strong case for moving from rigid, net-zero staffing plans to more flexible models that incorporate real-time adjustments.
How Modern Data Analytics Empowers WFM Teams
Rather than spending hours manually sifting through PDP reports, WFM teams can now use modern data analytics to uncover insights quickly and at scale. Tools like Python and other analytics platforms enable teams to rapidly process large datasets, identifying patterns of overstaffing and understaffing and quantifying the cost of these inefficiencies.
The goal here isn’t to simply automate the analysis itself (though that’s helpful)—it’s to use data-driven insights to show how legacy staffing models leave gaps in coverage or create excess. By analyzing staffing data over hundreds of intervals, WFM teams can craft a compelling narrative around the financial impact of sticking with traditional methods and the operational benefits of adopting more robust models.
“Staffing Levels Throughout the Day”
In the above chart, we can see staffing levels plotted across the day. The blue line represents scheduled staff, the red line shows the actual required staff, and the green line reflects actual staffing. The shaded red areas indicate Service Level Risks (understaffing), while the shaded green areas highlight Expense Risks (overstaffing). This type of analysis tells the story of how traditional models are prone to periods of inefficiency, either by not having enough agents to meet demand or by having too many agents during low-demand periods.
These insights are crucial for making the case for modern, real-time staffing models like Erlang-O, which factor in natural variance and volatility throughout the day.
Using PDP Data to Redesign Your Staffing Approach with Erlang-O
PDP data doesn’t just highlight inefficiencies—it offers a path to break free from the endless cycle of constant manual adjustments and reactive planning that many WFM teams face. By analyzing these reports, you can see exactly where your traditional staffing model—based on rigid, net-zero planning—falls short. Erlang-O provides a dynamic alternative by redesigning your staffing approach to integrate productive off-phone activities, such as training and coaching, directly into the staff line itself.
Instead of relying on a “buffer” of extra agents, Erlang-O incorporates planned, productive off-phone time—ensuring your team can respond to real-time changes without creating inefficiencies. This model accounts for the natural variance and volatility in call volumes and agent availability, allowing you to seamlessly reallocate agents based on actual demand.
For example, agents can be dynamically shifted to handle unexpected call spikes or moved to off-phone tasks during slower periods—all without the scrambling or service-level failures that plague legacy models. By embracing Erlang-O, you can build a staffing model that adapts in real-time, ensuring operational efficiency and consistent service levels, no matter how the day unfolds.
Unlock the Full Potential of Your PDP Data
PDP data and Python offer a powerful approach for identifying exactly where your current staffing model falls short. By analyzing it, you can clearly pinpoint inefficiencies like understaffing that leads to service-level failures or overstaffing that inflates costs.
We invite you to explore how simple analytics can reveal these insights and transform your workforce management strategy. See a live demonstration of how over/under analysis can be performed at scale using your own PDP data.
And this isn’t just about making adjustments to forecasts. It’s about using your own data to make smarter, real-time staffing decisions. The data is already in your hands—let’s unlock its full potential and build a more cost-effective, customer-focused operation. Get your complimentary Intraday Staffing Efficiency analysis, where we’ll help you quantify the inefficiencies baked into traditional staffing models and show how adopting a more dynamic, real-time approach—like Erlang-O—can improve both service levels and operational costs.
Contact us to learn more.
Learn how contact center automation solutions can improve your teams’ performance.
Fill out the form and we’ll be in touch.