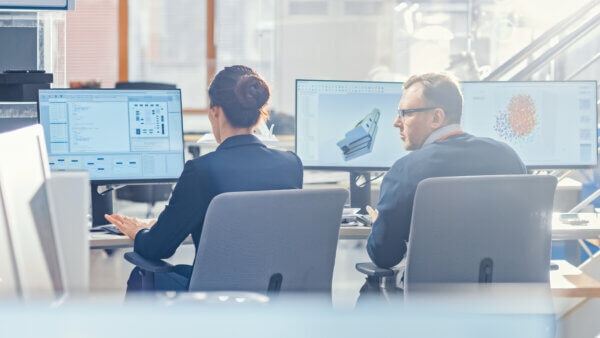
What Should We Expect From AI?
By Michael Jarus—Chief Architect, Intradiem
AI can solve problems we can think of, but it can’t think of problems we should solve.
Artificial intelligence (AI) is slowly but steadily transforming the way we do nearly everything, from driving cars to creating award-winning art. Half of all companies now use AI in at least one business function, and while many jobs have been replaced by machines, AI is expected to create millions of new jobs and inject up to $1.8 trillion into the economy by 2030. It’s the defining technological concept of our time.
Rooted in the scientific method, artificial intelligence uses a model-based approach to solving problems: observing facts, formulating hypotheses, testing them, analyzing the results, and drawing conclusions. The viability of this approach depends on how much data can be brought to bear on a problem, with a larger quantity of data generally yielding more reliable results.
For years, limits on the technical capacity to amass and analyze data have prevented organizations from mobilizing and processing enough of it to solve more than the simplest problems. But in recent years, a massive increase in computing power has made data more accessible and raised the roof of AI’s power to process and analyze raw information, resulting in an explosion of algorithms that control and facilitate many aspects of modern life.
For example, AI is integrated into image recognition programs used to assist in medical diagnoses. Facial recognition technology helps casinos, sports stadiums and other public venues identify known scammers and criminals. AI is woven into quality assurance (QA) processes to detect flaws in manufacturing processes. It’s used to recognize and assess the tendencies of athletes. The list of applications grows longer each day.
How Does AI Work?
Rooted in the scientific method, artificial intelligence uses a model-based approach to solving problems: observing facts, formulating hypotheses, testing them, analyzing the results, and drawing conclusions. The viability of this approach depends on how much data can be brought to bear on a problem, with a larger quantity of data generally yielding more reliable results.
Is AI About Intelligence or Velocity?
A massive expansion of computing power is allowing companies to harness unprecedented quantities of data, making AI the high R&D priority it is for so many organizations today. Just a generation or two ago, it would have taken several lifetimes to process the amount of data we can now process in just a few hours. In fact, data processing capacity today has expanded so much that an ordinary laptop could handle the 1969 moon launch.
But is that intelligence or just velocity? Computers can certainly “think” (process) much faster than humans, but they can’t do anything at all unless humans first tell them what to think. Some organizations go astray here, fixing their attention on execution but leaving out purpose, feeding mountains of data into sophisticated programs and expecting them to spit out something significant.
AI can solve problems we can think of, but it can’t think of problems we should solve. Reaching useful conclusions depends on asking the right questions and applying the scientific method. Artificial intelligence is used to detect genetic and other biological anomalies, for example, but without specific human instructions to pursue specific genetic markers, the process will return nonspecific (and therefore not very useful) results. If the program is trained to recognize specific genetic markers, on the other hand, it will do so accurately and thoroughly. All discovery is useful, of course, but directed discovery will always be more fruitful than random.
How to Hire for AI-Based Jobs
AI also represents a massive field of new career opportunities for technology professionals. Up-and-coming software engineers interested in working in AI and its sub-fields (machine learning, deep learning, natural language processing, robotics and others) may presume they need a whole new set of skills, but that’s not the case. A successful AI software engineer still relies on the core skills that engineers have always used to convert abstract ideas into useful tools. These skills include the ability to think analytically, reason abstractly, avoid bias, and express complex concepts in ways that non-technical people can understand.
Like others, my company is currently working to incorporate more AI capabilities into our product offering. We’ve had success hiring young software engineers, and I believe that’s because new engineers are generally free of bad habits, blind spots, or limits they may have picked up from previous employers. An engineer who’s works for years may develop rigid ideas about what works and what doesn’t.
Take the QA function in manufacturing I mentioned above, for instance: If an engineer spends ten years in an organization that accepts a 1.2 percent product defect rate as acceptable, for instance, that engineer may be less motivated to push through obstacles to achieving the 0.7 percent defect rate tolerance demanded by a different employer.
Being an experienced engineer has its advantages, of course. Seasoned engineers are generally better at collaborating and have learned the critical lesson that real companies work within time and budget limitations—that it’s not always possible to explore endless theoretical paths to achieve an outcome. As a student, gaining experience is the point, but in a real company, we need to achieve results.
In general, I look for engineers who have a clear understanding of their own personal limitations. Regardless of experience level, I find that people who are a bit unsure of themselves often make the best engineers because they tend to question their assumptions automatically. It’s OK to struggle and even fail, as long as you openly acknowledge failure and these mistakes help the team identify and eliminate false paths to finding the best solution for a given problem.
Communication skills are often overshadowed by technical prowess, but they really are critical. If you have a great idea but can’t make other people whose input is necessary to bring that idea to life understand it, your idea will never gain any traction. For example, if I were to come up with a cure for cancer but couldn’t explain it to other experts necessary to bring it to practical application, no one would be cured.
Changing the AI Game
Artificial intelligence is a game-changer, but it’s also a fairly predictable next step in the long quest to harness technology for improvements to human life. It gets closer to mimicking human intelligence every day, but it will never be able to take over and turn against us. AI is not destined to substitute for people; it’s intended to help us do a better job at solving problems that prevent us from living and working up to our full potential.
And as long as it’s driven by quintessentially human skills — curiosity, skepticism, reason — artificial intelligence will continue to knock down barriers and ensure that the only limits we face as humans are the limits of our own imagination.
This post originally appeared on builtin.com.
Back to Blog